Purpose:
This outline summarizes the implementation of using technology to improve safety in the workplace.
Executive Summary:
The implementation of Human and Operational Performance (HOP) in conjunction with Behavior Based Safety (BBS) can be greatly augmented by utilizing technology. This paper outlines the concepts to be used to realize improvements in safety by making careful and well thought out use of present and future technologies as they become available.
Human and Operational Performance (HOP) is a process that helps to shift blame away from the worker and helps to identify how we can make the workplace an environment where when the worker errs, that they err more safely. It is labor intensive to identify every area in the workplace that can pose hazards to the worker(s). Once those areas are identified, it is also time and cost intensive to make changes to those areas in the workplace that fall in line with HOP’s core principles.
HOP’s guidelines:
- Human error is normal
- People make mistakes and blame fixes nothing
- Blame fixes nothing
- We can predict, manage, and prevent error-likely situations by creating safeguards and the capacity to fail safely
- Learning is vital
- Organizations influence individual behavior
- Context drives behavior
- Violations of rules are rarely intended to cause harm and often make sense at the time
- How to respond to failure matters
- How leaders respond to failure matters
Behavior Based Safety (BBS) is an approach to workplace safety that focuses on identifying and modifying unsafe behaviors to prevent accidents and injuries. Here are the key aspects of BBS:
- Behavioral Focus: BBS emphasizes that most workplace accidents are caused by unsafe behaviors rather than unsafe conditions. By observing and analyzing these behaviors, organizations can identify patterns and areas for improvement.
- Observation and Feedback: The process involves regular observation of employees’ behaviors, followed by constructive feedback. This helps workers become aware of their actions and encourages safer practices.
- Employee Involvement: BBS relies on active participation from employees at all levels. Workers are encouraged to observe each other and provide feedback, fostering a culture of safety and mutual accountability.
- Data-Driven: BBS uses behavioral data to track progress and identify trends. This data helps in making informed decisions about safety interventions and measuring the effectiveness of safety programs.
- Continuous Improvement: The approach promotes ongoing assessment and refinement of safety practices. By continuously monitoring and addressing unsafe behaviors, organizations can create a safer work environment.
Implementing BBS can lead to a significant reduction in workplace accidents and injuries, creating a safer and more productive work environment.
BBS’s guidelines:
A proactive approach to increasing workplace safety
- By observing individual behavior
- Analyzing consequences
- Reinforcing desired safety behaviors
As the project involves improving Safety in the Workplace using technology, the use of AI will have, but not be limited to, multiple implementations and applications.
The overall strategic plan for implementation of AI technology, including a combination of AI technologies involves the following:
- Introduce AI in a building-block or “LEGO” approach. Start with a smaller objective or project and include personnel whose roles will be affected by the implementation.
- Safety personnel, Subject Matter Experts (SMEs) design engineers, and outside resources as needed.
- Having gained acceptance of the first implementation by those whose roles are affected, utilize their acceptance in helping to build upon the next building-blocks. Word-of mouth by those enjoying the effectiveness of the initial project can be invaluable.
- Continue expanding the implementation of AI technologies with subsequent projects that work well with previous successful implementations, where possible and practical.
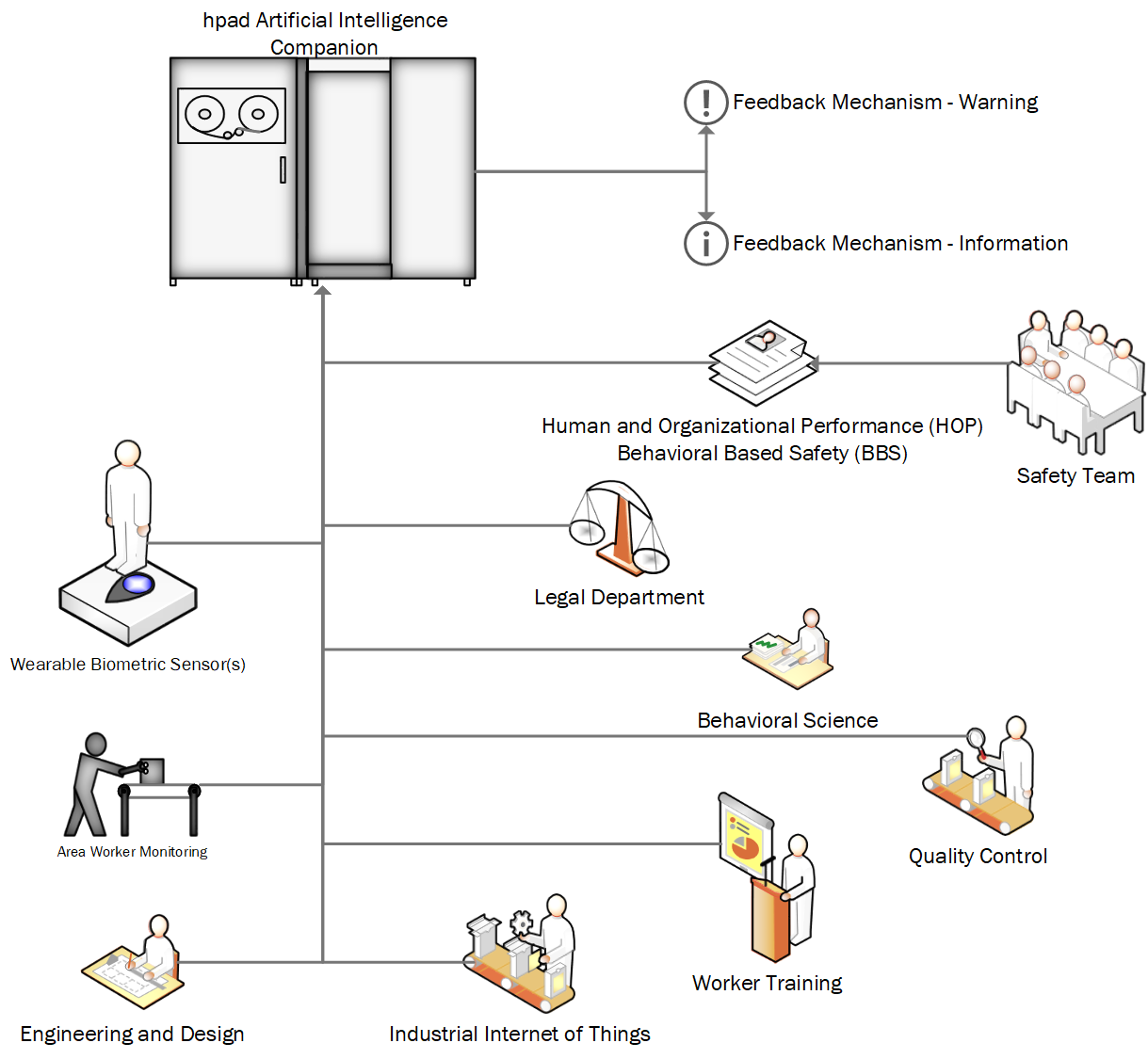
Figure 1 – Representation of overall project.
As there will be multiple implementations of AI, under several segments of the overall project, it is possible to make use of more than one strategy outlined by Michael Porter.
- Cost Leadership (Generic strategies as per Michael Porter)
- AI in safety can help to reduce workplace accidents resulting in a cost reduction in worker lost time, insurance costs, and potential fines.
- Cost leadership can be realized as the implementation of the AI technology will be a holistic approach. This means that as the AI learns its environment numerous strategies can be assessed for efficacy. Among the advantages are:
- Faster and more accurate identification of First Principles analysis of work cells.
- Cost analysis for area improvements in safety.
- Behavior analysis for improving worker behaviors within the workplace.
- Monitoring of safety regulatory standards including whether those standards apply and are being utilized effectively.
- More effective forensic analysis, with the added data from the previous and ongoing analysis of the work cells to better help improve the area(s) for HOP’s goals.
- Differentiation (Creating uniquely desirable products and services as per Michael Porter)
- Utilizing AI differentiates the company from others by having a better understanding of workplace hazards in more of a real-time basis, along with the analysis of historical data to help build a better and safer work environment.
- Focus (Offering a specialized service in a niche market as per Michael Porter)
- Utilizing a holistic approach to Safety in the Workplace, with AI as a key constituent, closely monitored by people, can provide a focused and clearer path to improving safety in the workplace.
The Current State of Technologies
The current state of technologies is not too dissimilar to many manufacturing-oriented companies. With the implementation of HOP and BBS, accident rates have been reduced but, unfortunately, still occur at a rate that is too high – one accident is too many. Though HOP and BBS aim to make the workplace safer by identifying areas that when a worker errs, that error can lead to a serious injury or worse. The identification of those areas and subsequently acting on that information is very labor intensive and time-consuming.
The current state of technologies used involves the following: (These solutions differentiate a company from many other manufacturing companies.)
- Typically, the identification and recording of accidents and incidents is carried out manually by keying information into specific fields in a database. This procedure is carried out company-wide by safety resources and is required to be done for any accident or incident.
- A Root Cause Analysis is performed when and where the accident or incident warrants it.
- This system can be used for a forensic analysis to be done at a later date.
- The information gathered by the system is used to help improve on HOP’s principles.
- A Safe Work Permit application can also be used when a maintenance event must be carried out. The application, through a list of questions and information, helps to identify hazards involved in the execution of the maintenance event. The maintenance worker(s) use this application to request a work order. Once the maintenance worker(s) have completed the process successfully, a work order is issued. The Safe Work Permit involves the following:
- General Job Information – Identify the exact location, date and time issued, contractor/dept., equipment, and job/task description. Sites can add additional information as needed.
- Specific Permits Required – Identify other specific permits required for this task, such as: hot work, line breaking, confined space entry, excavation, energized electrical work, critical lift, or crane suspended personnel basket.
- Job Specific Hazards – Consider the potential hazards that are likely to be present based on the work location or job to be done. If hazards exist, explain how the hazards will be controlled.
- Procedures, Personal Protective Equipment (PPE), and Equipment – Identify workplace procedures, PPE, equipment, and other controls that are needed to manage the risks of the permitted work. Note that PPE requirements are generally based on the site’s PPE Hazard Assessment.
- The utilization of a maintenance worker application is another proactive approach to increasing safety in the workplace.
Proposed initiative
The following AI technologies will be utilized in the overall goal of increasing safety in the workplace.
Initial AI Module Implementation - Supervised Learning – Safe vs Unsafe Areas and Practices
Utilize machine learning to enhance the overall goal of HOP and BBS to make the work environment such that when a worker errs, that they err safely.
This involves the identification and or creation, where currently lacking, of data sources necessary to analyze and monitor the work environment. Hazards, both in the specific and overall architecture of the work cell(s), and processes carried out by the worker(s). These hazards and processes will be thoroughly scrutinized and identified with respect to safety. First Principles will be utilized to determine best practices for each work cell. This scrutiny will be carried out by a supervised learning AI agent. The findings by the AI agent will also be analyzed by Subject Matter Experts (SMEs) for accuracy.
Processes will be re-evaluated for safety based on the findings from the AI agent, in conjunction with and under the tutelage of the SMEs. Where and when possible, work cells will be reworked under the criteria identified to make them safer for the worker(s).
Studies will need to be performed to better understand the workers’ interaction with their specific work cell(s). These studies will be designed to gather the following:
- Normal or desired behavior within the work cell.
- Undesired behavior within the work cell.
- Nuanced behaviors that are acceptable but specific to each worker within their work cell.
- Nuanced behaviors that are unacceptable or potentially dangerous but specific to each worker within their work cell.
- Are the current worker behaviors and interaction with the machine(s) the ideal, considering First Principles?
Second AI Implementation - Machine Learning Formulation – Work Cell Hazard Identification
Utilized to build on the supervised learning module described above to help analyze the work cell for potential hazards and recommend changes to that work cell to minimize or eliminate those hazards to the worker, a combination of human and machine learning.
The machine learning system will be fed historical and existing data from the company in the form of natural language, written guidelines and procedures, safety incident(s) data, and from safety organizations including, but not limited to – OSHA and ISO - ISO 45001 for example.
Humans will also be required to work in conjunction with the machine learning to assure optimum and more importantly, valid solutions to workplace hazards.
Third AI Implementation - Supervised Learning – Work Cell Implementation
Utilize machine learning to monitor and analyze worker(s)’ movements within a work cell, using skeletal tracking. The worker(s)’ movements would be monitored for the following:
- Proper work cell functions.
- Is the worker following the prescribed steps in an orderly manner?
- Is the worker exhibiting behavior that can indicate loss of attention or cognition?
Machine Learning Formulation:
- Skeletal tracking using – Cubemos, utilizing Intel’s RealSense™ computer vision facilitates the identification of the workers’ major skeletal positioning in space in real-time, along with location awareness. This system can be taught proper behavior and can then alert when and if the proper behavior is not followed.
- This skeletal tracking system will need to be developed for on-site deployment.
- There is enough regularity in proper behavior, and when improper, or chaotic behavior is detected, the AI agent can detect the undesired behavior.
- The machine learning algorithms would be supervised as the proper behaviors and paths would be mapped and cataloged and the worker(s)’ behaviors and motions would be compared to the ideal.
- When a worker’s behavior is determined by the AI agent to be dangerous or outside the guidelines of proper and trained actions, the system will “nudge” the worker, providing an early warning.
Fourth AI Implementation - Supervised Machine Learning – Maintenance Worker Implementation
Worker(s)’ cognition or attention to the task(s) at hand would lend itself to a machine learning application or implementation.
This system would be employed for maintenance activities, both - and initially – for regular maintenance tasks but also for irregular maintenance activities after the AI agent becomes more competent in its analysis. By monitoring the actions, both through their interaction with the machinery and with skeletal tracking, it is possible to capture when the worker’s attention wanders away from the task(s) at hand and then act accordingly by providing feedback in the form of “nudges” to the worker to alert them of their behavior(s).
Studies on human behavior would help to provide baseline data on attentiveness and also, potentially, when and where a worker loses focus.
Initial implementation of this scheme could replicate the exchange NASA Mission Control has with an astronaut performing a spacewalk. As safety of the astronaut is paramount, every step in the spacewalk is verbalized and acknowledged. This scheme lends itself well to an exchange between a maintenance worker and the AI natural language processor, along with other “Lego” building blocks to assure proper maintenance steps and ultimately alert when the worker has missed a step, for example, before the worker misses that step.
IT Considerations
Within all the above initiatives, it will be necessary to analyze the work cells and areas for connectivity. It is necessary to have a high data throughput capability for the amount(s) of data that will be captured. This includes any feedback to the worker(s) needed by the AI agents. It is anticipated that the early adoptions of these initiatives will be utilizing edge computing topology. This will support the speeds necessary for the AI agents to act upon and react quickly enough to be effective.
Leadership Considerations
With the oversight, direction, and responsibility of the innovation team, department heads and SMEs will be required to participate, including the responsibilities stated earlier, in the initial conception, initial testing, and subsequent roll out of each implementation. Those affected by the implementation will be a part of the design phases, testing, and roll out as well.
With full participation from various levels within the company, everyone will be more familiar with the system. Acceptance by ownership is the goal with this methodology.
The Advantage
The implementation of these technologies will differentiate the company from others by helping to revolutionize safety in the workplace. Mistakes can be reduced, quality can be improved, and most importantly – lives can be saved.
Plan of Action
The timeline and milestones for implementation are as follows:
- Begin the process of implementation with a Workshop.
- The Workshop will help to identify the project scope to all parties affected.
- The Workshop will include all those affected by the implementation of this system.
- We have found that to gain acceptance by every department affected by this project, it is important to involve at least one representative from each department in the Workshop.
- Details of the Workshop will be presented far enough in advance of the scheduled Workshop to allow those invited to detail their areas of interest, including any concerns they may have.
- Initial AI Module Implementation – Supervised Learning – Safe vs Unsafe Work Areas and Practices – Note: The hpad AI Companion™ has the unique ability to grow and expand to every implementation of the different aspects of Artificial Intelligence. This unique ability proves to be a huge cost advantage for the end user(s).
- Assemble the team required to design and build the prototype implementation – This follows for each implementation and requires a hands-on approach from all involved
- Innovation Team members – Technologists
- Responsibility lies within this group
- AI programmers
- Accountability lies within this group
- Subject Matter Experts – Internal company SMEs
- This group will be consulted for their knowledge and expertise in the operation of the machine(s) and production, including safety SMEs
- Stakeholders – Those responsible for their areas of manufacturing and production
- This group will be informed about every process in this endeavor
- This group will also be responsible for participating in each implementation as it directly applies to their area(s) of expertise
- Data and documentation to be consumed by the AI agent:
- Gather historic safety incident data
- Concentrate on one area in one plant location:
- Gather machine engineering drawings
- Gather process flow / recommended best practices for the workers
- Gather existing machine data if available
- If machine data is lacking, correct that by building in necessary data sources
- Create supervised learning AI agent to consume all of the above information and data
- SMEs to work with the AI engineers with the goal of having the AI agent focus on establishing what safety of the area and the worker(s) is and what work cells and processes need attention to satisfy Human and Organizational Performance (HOP) principles.
- Prove the concept under these conditions
- Have the SMEs and stakeholders evaluate the findings for efficacy
- Machine Learning Formulation – Work Cell Hazard Identification
- Expand on what was learned in the initial implementation
- Include the following to be consumed by the AI agent:
- Gather safety and best practices from the following:
- ISO, OSHA, NFPA, etc.
- Internal safety documentation – HOP
- SME’s best practices
- Historical data related to incidents and accidents associated with that process or area
- Create a machine learning AI Natural Language Processor (NLP) to consume the above with the goal of identifying unsafe work cells, unsafe or hazardous processes.
- Train the AI to take those processes down to the first principles concept.
- This will help to view those areas and processes in a different light.
- With the help of the SMEs, act on those areas and processes that have been identified as unsafe.
- Supervised Learning Implementation – Work Cell Implementation
- Continue building on the above implementations by adding the following:
- Establish in the AI agent the following, including functionality established in the initial implementation:
- Introduce Skeletal tracking to the overall scheme
- Teach the AI what proper and improper behavior in a work cell is
- Map those behaviors in the AI
- Generate algorithms in the AI to act accordingly when there are deviations from proper behavior
- Develop when and how a worker is to be “nudged”, safely, when their behavior is found to be improper or unsafe
- Prove the concept under these conditions
- Have the SMEs and stakeholders evaluate the findings for efficacy
- Supervised Machine Learning – Maintenance Worker(s) Interaction
- Continue building on the above implementations by adding the following:
- Monitor the maintenance worker(s) behavior through a back-and-forth dialog between the worker(s) and an AI NLP
- Target the worker(s) cognition with methods in the NLP that are aimed at recognizing deviations in the worker’s cognition level.
- SMEs will closely supervise and monitor the exchange(s) between the maintenance worker and the NLP and intervene when and where necessary, until which time the AI NLP becomes mature enough to be left to its own.
- Those interventions will also be cataloged for retraining and updating of the AI NLP algorithms.
- Start with regular and more simplistic maintenance tasks
- Once efficacy has been established and proved, expand the system’s influence with more irregular maintenance tasks
- Continue to build upon and optimize the AI and expand when efficacy has been established in each implementation
The area of safety brings along with it the critical responsibility of safety of the workers. Mistakes and false positives generated by the AI agents will need to be kept to a minimum, or if possible, eliminated altogether. False positives can potentially lead to an accident at worst, and lead to a level of distrust by those the system is designed to protect. Extreme care must be taken to assure this is covered to every extent possible.
There are also concerns about the identity of those workers being monitored for their actions while at work. Every effort will be taken to assure any data gathered cannot be traced to the individual.
The implementation of this technology can lead to expanded responsibilities of those roles currently within the safety departments. Safety personnel’s time can be freed up from manually analyzing the large amounts of data to allow them to migrate to working alongside the AI agent(s). Their experience can also be utilized in helping to establish new and safer work processes and better and safer work cells.
Success will be measured in fewer accidents and incidents in the workplace.
Timeline:
The timeline is to be determined based upon the complexity of the initial implementation. Typically, an implementation can take between three and six months. More complex projects can be expected to take more time.
Typical implementation will consist of the following:
- Identification of the scope of the project.
- Identification of all departments affected by the project.
- It is advantageous to include all departments affected by this implementation for best acceptance by all involved.
- Generation of the “stack’ necessary for the hpad AI Companion to exist.
- Gathering of SME’s knowledge into the hpad AI Companion.
- Gathering of any existing documentation relative to the project scope and environment for consumption into the hpad AI Companion.
- Training of SMEs to interact with the hpad AI Companion.
- Behavioral analysis of those working in the environment identified by the project scope and purpose.
- Both positive and negative behaviors will be monitored and analyzed
- Testing and validation by the SMEs of initial and subsequent results generated by the hpad AI Companion.
- This builds a level of confidence in the results of the Companion such that testing and validation can become less frequent.
- This also helps to build expertise in not only the querying and interaction of SMEs with the hpad AI Companion but also provides opportunities in SMEs developing new ideas through a better viewpoint to add to the corpus of the overall project and environment.
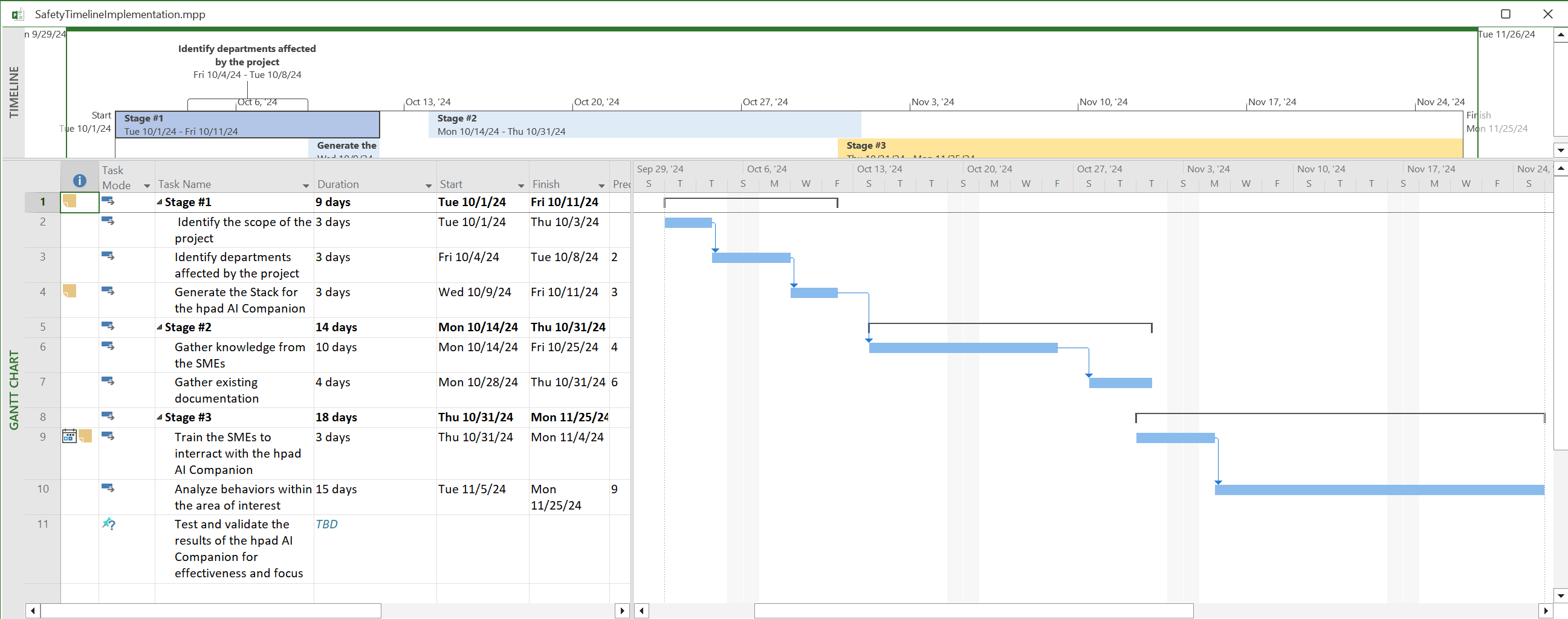
Figure 2 – Example timeline for implementation.
Conclusion:
Chaotic Solutions, LLC, concludes that the current level of safety incidents throughout industry can be reduced by utilizing technologies including but not limited to the following:
- Artificial Intelligence
- Smart Wearables
- Video surveillance powered by Artificial Intelligence
- First Principles
- In philosophy and science, a “first principle” is a basic proposition or assumption that cannot be deduced from any other proposition or assumption.
Appendix / References:
Michael Porter:
The Competitive Advantage - https://www.mindtools.com/pages/article/newSTR_82.htm
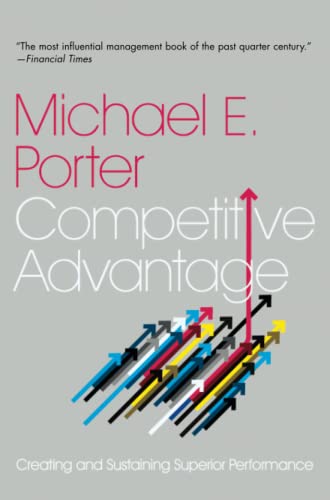
ISBN-13: 9780684841465
Michael Porter called the generic strategies "Cost Leadership" (no frills), "Differentiation" (creating uniquely desirable products and services) and "Focus" (offering a specialized service in a niche market). He then subdivided the Focus strategy into two parts: "Cost Focus" and "Differentiation Focus." These are shown in figure 1 below.
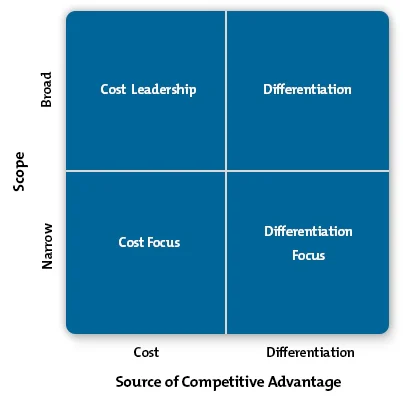
Figure 1- Porter's Generic Strategy Model
Michael Porter’s generic strategies are a framework for achieving competitive advantage in the market. They include three main strategies:
- Cost Leadership: This strategy focuses on becoming the lowest-cost producer in the industry. Companies achieve this by optimizing their production processes, reducing costs, and offering products at lower prices to attract price-sensitive customers.
- Differentiation: This strategy aims to offer unique products or services that stand out from competitors. Companies focus on innovation, quality, and features that appeal to customers who are willing to pay a premium for something distinctive.
- Focus: This strategy targets a specific market segment or niche. Companies can either pursue cost focus (offering lower prices within the niche) or differentiation focus (offering unique products tailored to the niche). This approach allows companies to serve the specific needs of a particular group of customers more effectively than competitors.
Porter emphasized that companies should choose one of these strategies to avoid wasting resources and to achieve a sustainable competitive advantage.
First Principles:
In philosophy, First Principles refer to the most basic and fundamental propositions or assumptions that cannot be deduced from any other propositions or assumptions. These principles serve as the foundational building blocks for any system of thought or knowledge.
Here are some key aspects of First Principles:
- Axiomatic Nature: First principles are self-evident truths that do not require proof. They are the starting points for reasoning and cannot be derived from other principles.
- Foundational Role: They form the basis upon which other knowledge is built. For example, in Euclidean geometry, the axioms and postulates are considered first principles.
- Philosophical Importance: In epistemology (the study of knowledge), first principles are crucial for understanding the nature and limits of human knowledge. They are often associated with a priori knowledge, which is knowledge that is independent of experience.
- Historical Context: The concept of first principles dates back to Ancient Greek philosophy. Aristotle, for instance, emphasized the importance of identifying first principles in his works on metaphysics.
First principles thinking encourages breaking down complex problems into their most basic elements and building up from there, ensuring that conclusions are based on fundamental truths.